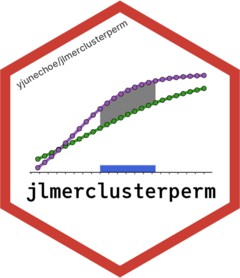
Calculate bootstrapped p-values of cluster-mass statistics
Source:R/calculate_pvalue.R
calculate_clusters_pvalues.Rd
Calculate bootstrapped p-values of cluster-mass statistics
Usage
calculate_clusters_pvalues(
empirical_clusters,
null_cluster_dists,
add1 = FALSE
)
clusters_are_comparable(empirical_clusters, null_cluster_dists, error = FALSE)
Arguments
- empirical_clusters
A
empirical_clusters
object- null_cluster_dists
A
null_cluster_dists
object- add1
Whether to add 1 to the numerator and denominator when calculating the p-value. Use
TRUE
to effectively count the observed statistic as part of the permuted null distribution (recommended with largernsim
prior to publishing results).- error
Whether to throw an error if incompatible
Examples
# \donttest{
library(dplyr, warn.conflicts = FALSE)
# Specification object
spec <- make_jlmer_spec(
weight ~ 1 + Diet, filter(ChickWeight, Time <= 20),
subject = "Chick", time = "Time"
)
spec
#> ── jlmer specification ───────────────────────────────────────── <jlmer_spec> ──
#> Formula: weight ~ 1 + Diet2 + Diet3 + Diet4
#> Predictors:
#> Diet: Diet2, Diet3, Diet4
#> Groupings:
#> Subject: Chick
#> Trial:
#> Time: Time
#> Data:
#> # A tibble: 533 × 6
#> weight Diet2 Diet3 Diet4 Chick Time
#> <dbl> <dbl> <dbl> <dbl> <ord> <dbl>
#> 1 42 0 0 0 1 0
#> 2 51 0 0 0 1 2
#> 3 59 0 0 0 1 4
#> # ℹ 530 more rows
#> ────────────────────────────────────────────────────────────────────────────────
# Make empirical clusters
empirical_statistics <- compute_timewise_statistics(spec)
empirical_clusters <- extract_empirical_clusters(empirical_statistics, threshold = 2)
empirical_clusters
#> ── Empirical clusters (t > 2) ────────────────────────── <empirical_clusters> ──
#> Diet2
#> [4, 8]: 8.496
#> Diet3
#> [2, 20]: 34.628
#> Diet4
#> [2, 20]: 39.371
#> ────────────────────────────────────────────────────────────────────────────────
# Make null cluster-mass distribution
reset_rng_state()
null_statistics <- permute_timewise_statistics(spec, nsim = 100)
null_cluster_dists <- extract_null_cluster_dists(null_statistics, threshold = 2)
# Significance test the empirical cluster(s) from each predictor against the simulated null
calculate_clusters_pvalues(empirical_clusters, null_cluster_dists)
#> ── Empirical clusters (t > 2) ────────────────────────── <empirical_clusters> ──
#> Diet2
#> [4, 8]: 8.496 (p=0.0200)
#> Diet3
#> [2, 20]: 34.628 (p=0.0000)
#> Diet4
#> [2, 20]: 39.371 (p=0.0000)
#> ────────────────────────────────────────────────────────────────────────────────
# Set `add1 = TRUE` to normalize by adding 1 to numerator and denominator
calculate_clusters_pvalues(empirical_clusters, null_cluster_dists, add1 = TRUE)
#> ── Empirical clusters (t > 2) ────────────────────────── <empirical_clusters> ──
#> Diet2
#> [4, 8]: 8.496 (p=0.0297)
#> Diet3
#> [2, 20]: 34.628 (p=0.0099)
#> Diet4
#> [2, 20]: 39.371 (p=0.0099)
#> ────────────────────────────────────────────────────────────────────────────────
# This sequence of procedures is equivalent to `clusterpermute()`
reset_rng_state()
clusterpermute(spec, threshold = 2, nsim = 100, progress = FALSE)
#> $null_cluster_dists
#> ── Null cluster-mass distribution (t > 2) ────────────── <null_cluster_dists> ──
#> Diet2 (n = 100)
#> Mean (SD): 0.266 (2.13)
#> Coverage intervals: 95% [0.000, 6.579]
#> Diet3 (n = 100)
#> Mean (SD): -0.124 (2.75)
#> Coverage intervals: 95% [-4.777, 5.707]
#> Diet4 (n = 100)
#> Mean (SD): 0.007 (2.50)
#> Coverage intervals: 95% [0.000, 0.000]
#> ────────────────────────────────────────────────────────────────────────────────
#>
#> $empirical_clusters
#> ── Empirical clusters (t > 2) ────────────────────────── <empirical_clusters> ──
#> Diet2
#> [4, 8]: 8.496 (p=0.0297)
#> Diet3
#> [2, 20]: 34.628 (p=0.0099)
#> Diet4
#> [2, 20]: 39.371 (p=0.0099)
#> ────────────────────────────────────────────────────────────────────────────────
# The empirical clusters and the null cluster-mass distribution must be comparable
empirical_clusters2 <- extract_empirical_clusters(empirical_statistics, threshold = 3)
# For example, below code errors because thresholds are different (2 vs. 3)
try( calculate_clusters_pvalues(empirical_clusters2, null_cluster_dists) )
#> Error in clusters_are_comparable(empirical_clusters, null_cluster_dists, :
#> Cluster-mass statistics between empirical and null are not comparable.
#> ℹ `empirical_clusters` and `null_cluster_dists` must share the same `statistic`
#> and `threshold`.
#> ✖ threshold: empirical uses 3 but null uses 2.
# Check for compatibility with `clusters_are_comparable()`
clusters_are_comparable(empirical_clusters, null_cluster_dists)
#> [1] TRUE
clusters_are_comparable(empirical_clusters2, null_cluster_dists)
#> [1] FALSE
# }