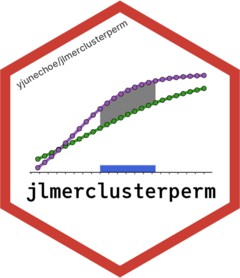
Construct a null distribution of cluster-mass statistics
Source:R/extract_clusters.R
extract_null_cluster_dists.Rd
Construct a null distribution of cluster-mass statistics
Arguments
- null_statistics
A simulation-by-time-by-predictor 3D array of null (permuted) timewise statistics.
- threshold
The threshold value that the statistic must pass to contribute to cluster mass. Interpretation differs on the choice of statistic (more below):
If
statistic = "t"
, the threshold for t-value (beta/std.err) from the regression model.If
statistic = "chisq"
, the threshold for the p-value of chi-squared statistics from likelihood ratio tests.
- binned
Whether the data has been aggregated/collapsed into time bins. Defaults to
FALSE
, which requires a cluster to span at least two time points. IfTRUE
, allows length-1 clusters to exist.
Examples
# \donttest{
# \dontshow{
options("jlmerclusterperm.nthreads" = 2)
jlmerclusterperm_setup(cache_dir = tempdir(), verbose = FALSE)
julia_progress(show = FALSE)
# }
library(dplyr, warn.conflicts = FALSE)
# Specification object
spec <- make_jlmer_spec(
weight ~ 1 + Diet, filter(ChickWeight, Time <= 20),
subject = "Chick", time = "Time"
)
spec
#> ── jlmer specification ───────────────────────────────────────── <jlmer_spec> ──
#> Formula: weight ~ 1 + Diet2 + Diet3 + Diet4
#> Predictors:
#> Diet: Diet2, Diet3, Diet4
#> Groupings:
#> Subject: Chick
#> Trial:
#> Time: Time
#> Data:
#> # A tibble: 533 × 6
#> weight Diet2 Diet3 Diet4 Chick Time
#> <dbl> <dbl> <dbl> <dbl> <ord> <dbl>
#> 1 42 0 0 0 1 0
#> 2 51 0 0 0 1 2
#> 3 59 0 0 0 1 4
#> # ℹ 530 more rows
#> ────────────────────────────────────────────────────────────────────────────────
# Null cluster-mass distributions are derived from the permuted timewise statistics
reset_rng_state()
null_statistics <- permute_timewise_statistics(spec, nsim = 100)
null_cluster_dists <- extract_null_cluster_dists(null_statistics, threshold = 2)
null_cluster_dists
#> ── Null cluster-mass distribution (t > 2) ────────────── <null_cluster_dists> ──
#> Diet2 (n = 100)
#> Mean (SD): 0.266 (2.13)
#> Coverage intervals: 95% [0.000, 6.579]
#> Diet3 (n = 100)
#> Mean (SD): -0.124 (2.75)
#> Coverage intervals: 95% [-4.777, 5.707]
#> Diet4 (n = 100)
#> Mean (SD): 0.007 (2.50)
#> Coverage intervals: 95% [0.000, 0.000]
#> ────────────────────────────────────────────────────────────────────────────────
# Collect as dataframe with `tidy()`
# - Each simulation contributes one (largest) cluster-mass statistic to the null
# - When no clusters are found, the `sum_statistic` value is zero
null_cluster_dists_df <- tidy(null_cluster_dists)
null_cluster_dists_df
#> # A tibble: 300 × 6
#> predictor start end length sum_statistic sim
#> <chr> <dbl> <dbl> <dbl> <dbl> <fct>
#> 1 Diet2 10 20 6 14.8 001
#> 2 Diet2 NA NA NA 0 002
#> 3 Diet2 NA NA NA 0 003
#> 4 Diet2 NA NA NA 0 004
#> 5 Diet2 NA NA NA 0 005
#> 6 Diet2 NA NA NA 0 006
#> 7 Diet2 NA NA NA 0 007
#> 8 Diet2 NA NA NA 0 008
#> 9 Diet2 NA NA NA 0 009
#> 10 Diet2 NA NA NA 0 010
#> # ℹ 290 more rows
# Changing the `threshold` value changes the shape of the null
extract_null_cluster_dists(null_statistics, threshold = 1)
#> ── Null cluster-mass distribution (t > 1) ────────────── <null_cluster_dists> ──
#> Diet2 (n = 100)
#> Mean (SD): 0.565 (5.52)
#> Coverage intervals: 95% [-9.681, 12.061]
#> Diet3 (n = 100)
#> Mean (SD): 0.089 (6.27)
#> Coverage intervals: 95% [-15.847, 12.185]
#> Diet4 (n = 100)
#> Mean (SD): 0.715 (5.23)
#> Coverage intervals: 95% [-7.843, 11.728]
#> ────────────────────────────────────────────────────────────────────────────────
extract_null_cluster_dists(null_statistics, threshold = 3)
#> ── Null cluster-mass distribution (t > 3) ────────────── <null_cluster_dists> ──
#> Diet2 (n = 100)
#> Mean (SD): 0.000 (0.00)
#> Coverage intervals: 95% [0.000, 0.000]
#> Diet3 (n = 100)
#> Mean (SD): 0.000 (0.00)
#> Coverage intervals: 95% [0.000, 0.000]
#> Diet4 (n = 100)
#> Mean (SD): 0.000 (0.00)
#> Coverage intervals: 95% [0.000, 0.000]
#> ────────────────────────────────────────────────────────────────────────────────
# \dontshow{
JuliaConnectoR::stopJulia()
# }
# }